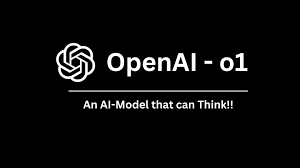
Artificial intelligence companies like OpenAI are entering a new era. Moving beyond simply scaling massive models, they’re pioneering smarter AI training techniques that prioritize human-like reasoning. This transformative shift could redefine AI research, hardware needs, and venture capital investments.
The End of “Bigger is Better”
For years, scaling large language models—powered by massive datasets and computational resources—drove AI advancements like OpenAI’s GPT-4. However, limitations such as diminishing returns, hardware failures, and global data shortages have emerged, forcing researchers to rethink their approach.
Ilya Sutskever, a leading AI researcher, described this evolution: “The 2010s were the age of scaling; now we’re back in the age of wonder and discovery.”
What Is Test-Time Compute?
OpenAI’s innovative test-time compute technique enhances AI performance during inference, the phase where models process real-world tasks. By dedicating more computing power to challenging problems in real-time, this approach mimics human decision-making and problem-solving.
OpenAI’s latest o1 model, built with test-time compute, showcases multi-step reasoning and expert-curated feedback. Researchers observed that allowing AI to “think” longer for complex tasks yielded results comparable to extensive model scaling.
Why This Matters for AI Hardware
This shift toward smarter AI training techniques has far-reaching implications for hardware. While Nvidia’s chips dominate large-scale training, the rise of inference clouds—distributed servers that power real-time model usage—might reduce demand for traditional AI chips.
Venture capital firms, which have heavily funded AI innovation, are paying close attention. “This transition moves us toward inference clouds,” said Sonya Huang of Sequoia Capital, signaling a change in how AI models will operate.
The Competitive Landscape
Leading AI labs, including Google DeepMind, Anthropic, and xAI, are also adopting these advanced techniques, intensifying the competition. OpenAI’s strategy, however, remains clear: to stay several steps ahead by continually refining its models and methods.
Conclusion: The Future of AI Training
The transition from scaling to smarter AI techniques marks a pivotal moment in the field. By prioritizing innovation over brute force, AI systems are becoming more efficient and capable of human-like thinking. As OpenAI and others lead this transformation, the industry is set to evolve dramatically in the coming years.