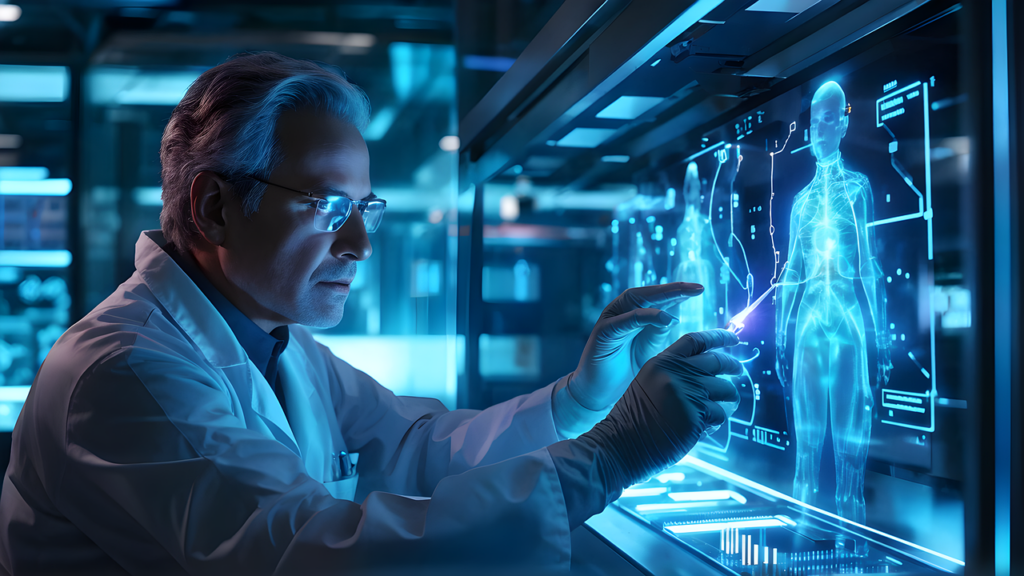
AI in bioengineering is transforming the way scientific data is generated, analyzed, and applied. With challenges like biased datasets and underrepresentation plaguing fields like healthcare and biomedicine, artificial intelligence offers innovative solutions to promote equity. By addressing systemic gaps in data collection and interpretation, AI has the potential to make scientific research more inclusive and reliable, ensuring better outcomes for all.
The Role of AI in Bioengineering
In bioengineering, equitable data is the foundation of innovation. However, achieving it is far from simple. Datasets are often:
- Biased or incomplete, reflecting systemic inequities in data collection.
- Sensitive, especially when tied to privacy concerns in patient health records.
- Unrepresentative, skewing results and excluding diverse populations.
These issues can lead to serious consequences, such as flawed research conclusions or ineffective treatments. For example, clinical trials heavily weighted toward specific demographics fail to account for broader patient needs, resulting in disparities in healthcare advancements.
How AI in Bioengineering Addresses Bias
Artificial intelligence, especially generative AI, is a game-changer for bioengineering. Here’s how it bridges gaps:
- Bias Detection and Analysis
AI systems can analyze datasets to uncover hidden patterns, revealing biases that might otherwise go unnoticed. This insight allows researchers to adjust their methodologies, ensuring more representative data. - Synthetic Data Generation
Generative AI can create synthetic data that mimics real-world datasets while addressing underrepresentation. For instance, models can simulate scenarios for populations often excluded from traditional research, like minority demographics or rare conditions. - Enhanced Accessibility
AI-driven platforms democratize bioengineering research, offering intuitive tools for data visualization and analysis. This improves access for researchers, organizations, and policymakers alike.
Synthetic Data: A Key Strength of AI in Bioengineering
One of the standout contributions of AI in bioengineering is its ability to generate synthetic data. Synthetic datasets:
- Bridge Data Gaps: By simulating diverse scenarios, they complement real-world data and expand representation.
- Model Rare Conditions: Adjusting parameters in AI models enables predictions and analyses of uncommon phenomena, critical in epidemiology and disease prevention.
Despite these advantages, synthetic data must undergo stringent validation. As highlighted in research published by Mihaela van der Schaar and colleagues in Nature, standard metrics are needed to ensure the reliability of AI-generated datasets. Without this validation, conclusions drawn from synthetic data may lack credibility.
Challenges and Opportunities
While AI in bioengineering shows promise, its reliance on real-world data introduces challenges. Biased training data can perpetuate inequities, raising concerns about trustworthiness. This is especially critical in healthcare, where algorithm-driven insights influence patient care.
To fully realize AI’s potential, researchers must tackle the root causes of data bias. Ethical frameworks, coupled with advancements in AI validation, are essential for fostering equity in bioengineering data.
Conclusion
AI in bioengineering has the power to revolutionize how data is generated and utilized. By addressing bias, enhancing accessibility, and enabling synthetic data creation, AI paves the way for more equitable and inclusive scientific research. However, achieving true equity requires a combined effort—balancing AI innovations with ethical and systemic reforms.
For more insights, explore the original research on Nature.